Poker AI: Statistical and Machine Learning Advances in artificial intelligence
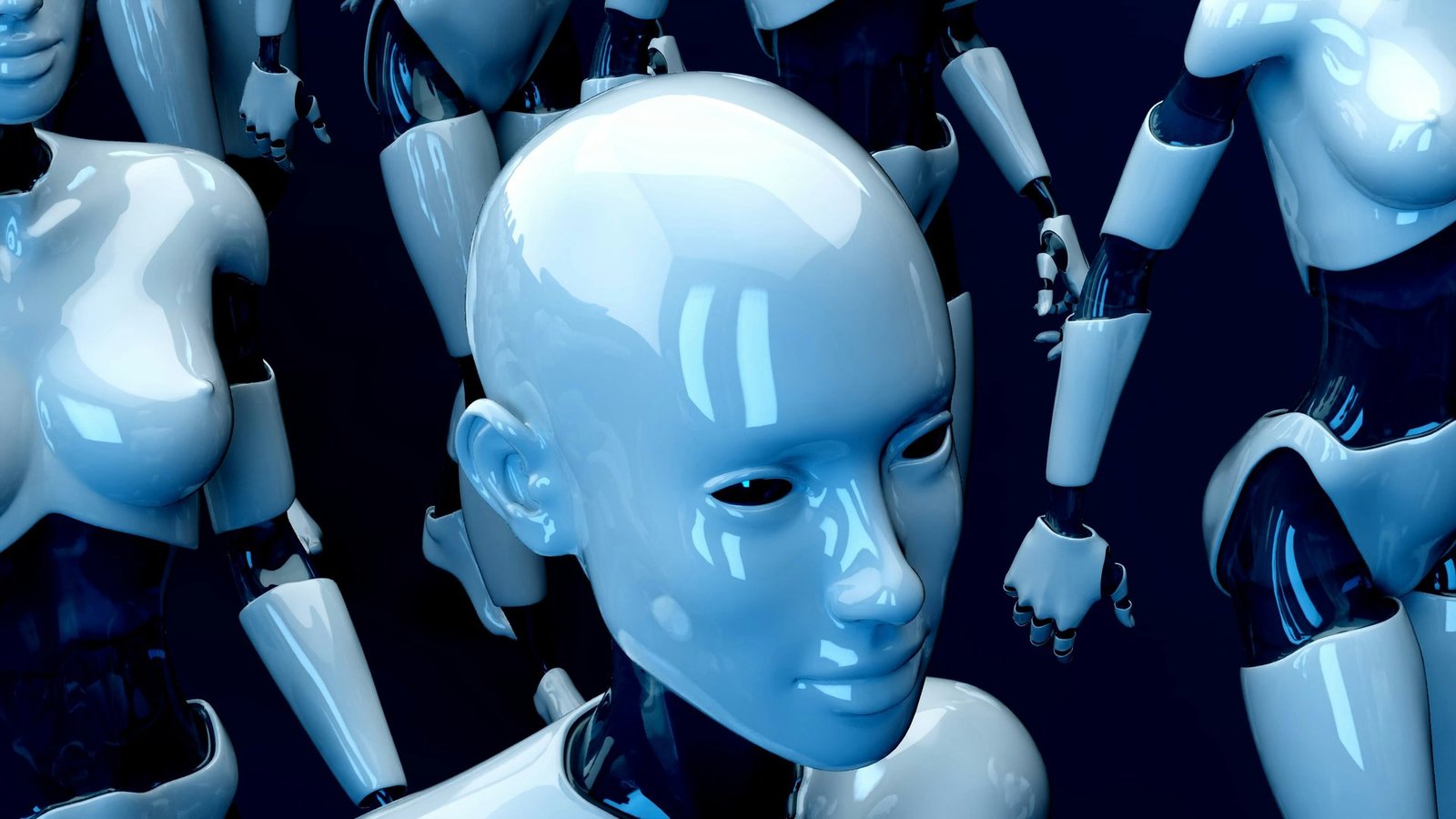
As poker went digital with the introduction of online poker rooms, the idea of poker bots was born. There had been a few attempts at computerized poker players as early as the 1980s, but online poker offered new opportunities. In the digital environment, if you have a poker bot that could play on its own, and beat human opponents, you could win money, and no one would know they were playing a bot at all.
Try NZT AI for free
Play in 18 supported venues, use table player level detector and autoclicker to scale your bot farm
AI Poker from Libratus to Pluribus
Those early bots didn’t use Artificial Intelligence as we know it today at all, instead relying on dedicated hardware and hardcoded rules systems to be able to ‘play’ the game. The results were not inspiring, and any human player with some experience in playing poker online could beat them easily. However, researchers kept going, and as AI developed, it was quickly adopted the technology as a way to automate poker play online.
At first, this was still restricted to hardware-based solutions, with Libratus, the most advanced of these, finally able to beat average human players on a regular basis. But the biggest jump came as AI technology made rapid advances, going from hardware-based to software-based algorithms that could be readily adapted to be used in AI poker bots.
That advance became Pluribus Poker AI and unlike Libratus, which required dedicated hardware, using a standard computer (the only major requirement was 128 GB of RAM), Pluribus took on a full table of professional poker players. Five humans, one poker bot, and Pluribus won. Not just once either, suddenly artificial intelligence could beat players at all levels, and do so reliably.
Adapting to Multiplayer Cash Game Dynamics
However, in this varied world of poker, even the mighty Pluribus AI poker bot was not the final answer. Playing tournament poker against five other players is a very different poker experience than playing cash games in an online poker room. In particular, cash games allow players to add more chips any time they want, so with variable stack sizes, there is a difference in strategy that changes the game significantly.
Here, in online poker cash games, AI systems cannot rely on baseline probabilities that reflect equal resources among all players, instead they face a constantly shifting approach that requires players, and AI poker bots, to adapt in real time to what is going in in a given hand or game. AI solutions like Libratus and Pluribus needed new strategies to perform well in games like no-limit texas Hold’em.
By necessity, that is a machine learning system that must be capable of reactive thinking to overcome those changes in opponent’s behavior, and how that plays out is the key to AI poker bot success.
Statistical approaches in poker
Statistics have been integral to poker analysis for many years, with top players using probability calculations to gain an understanding of likely outcomes at each turn. Although not technically a bot, virtual poker assistants have grown in popularity too, providing statistics of players and potential outcomes on screen in real time during an online poker game.
Perhaps the most famous use of statistics in poker is what is known as expected value, or EV. This concept calculate the potential of each hand, including both losses and wins, allowing you to make the right choice of whether to bet, raise or fold.
Combining EV with other data allows a poker bot AI to adopt what is known as a Game Theory Optimal (GTO) strategy. The idea is that GTO maximizes your performance in every hand. That means minimizing losses while making the most of winning situations. Poker AI that can maintain GTO strategies are the ultimate in automated poker play.
Handling Incomplete Information and Bluffing
But even with GTO strategies, there is one area where statistics are not the only answer. Poker is a game where there is incomplete information, that is, you don’t know what cards the other players have. This is what makes poker such a challenge, and why AI researchers like to use it to develop their systems.
That unknown information is then multiplied by another aspect of the game. Players don’t have to respond logically. A poor hand can become a winning hand through bluffing, but you don’t really know when a player is bluffing either. That is two lots of information that a player, including poker AI, will not have. This is the real challenge of poker beyond the statistics, and where the skill of player makes the difference. It is also the most difficult aspect of the game for Poker AI to master.
It can be done, Pluribus adopted a bluff detection algorithm to try and identify when players were bluffing, based around changes in player strategies and previous bluff history of that data is available. This proved relatively successful, and along with its own bluff routines, provided a more human approach to the game. As we see poker AI continue to advance, this is one area where there is plenty of room for improvement though.
Application of algorithms in the real world
Today’s poker AI algorithms appear in a number of tools. Bots themselves offer automated play and the ability to run on multiple accounts simultaneously. This means playing several tables at once, which even on micro stakes games, can offer a monthly income for the operator. Because they work without human input, these types of bot are increasing in popularity for those looking for a side hustle to earn money from online poker.
But that is not all, virtual poker assistants using AI algorithms offer a similar real-time game analysis, but instead of playing the game themselves, work to help you play more effectively. Analysis and suggestions appear on the screen, so unlike a bot you do play each hand yourself, but have the same analysis structure to help you make the most effective strategy choices during the game.
Finally, AI powered training solutions use the strength of AI performance to help players learn and understand best approach scenarios. Whether it is new players learning the game or professional players looking to refine particular aspects of their game, these AI powered training tools are another way in which machine learning and AI are influencing poker.
Machine learning in poker
We can see then, that machine learning processes are integral to the development of a range of tools that help players learn and win in online poker. As machine learning evolves, as has been the case over the years, it will help poker bots deliver improved performance, offer more nuance in terms of strategy choice, and be better at both recognizing and implementing bluffs.
There is a reason why so many researchers use poker for AI and machine learning development. It offers challenges that require true adaptability and understanding to master, and as machine learning advances, so do the tools that use it.
Conclusion
Machine learning and AI algorithms have had a prolific impact on online poker. From those early bots to the ability to beat professional players, advancements in machine learning technology and AI algorithms has seen poker bots and other similar tools leap forwards in capabilities.
Today, bots can be left to play games autonomously, don’t require any specialized hardware, and can play multiple tables at once, while delivering winning performances. However, that doesn’t mean they have peaked. Far from it, from refining the approach to unknown information and bluffing to more human-like behaviors to avoid bot detection systems, there is still many ways in which AI advancement can improve the poker experience.
FAQ
What statistical methods are used in poker?
There are several, although the most common is EV, or expected value, as a way to assess the potential of any given hand. Overall, statistical play tries to achieve the game optimal strategy, which minimizes losing hands and maximizes winning positions.
How does machine learning help create more effective poker strategies?
By getting better at understanding the nuances of game strategy, particularly the unknown aspects of the game, where statistical analysis cannot provide all the answers, in particular, player bluffs and the hidden card factors.
Which machine learning algorithms are most effective in analyzing poker game situations?
Pluribus is the most effective algorithm out there, capable of beating multiple professional players simultaneously.
Can statistical and machine learning techniques help players with less experience improve their game?
Yes. Either within a dedicated training tool, or as a virtual poker assistant that helps players understand strategies and actions more clearly during a game.